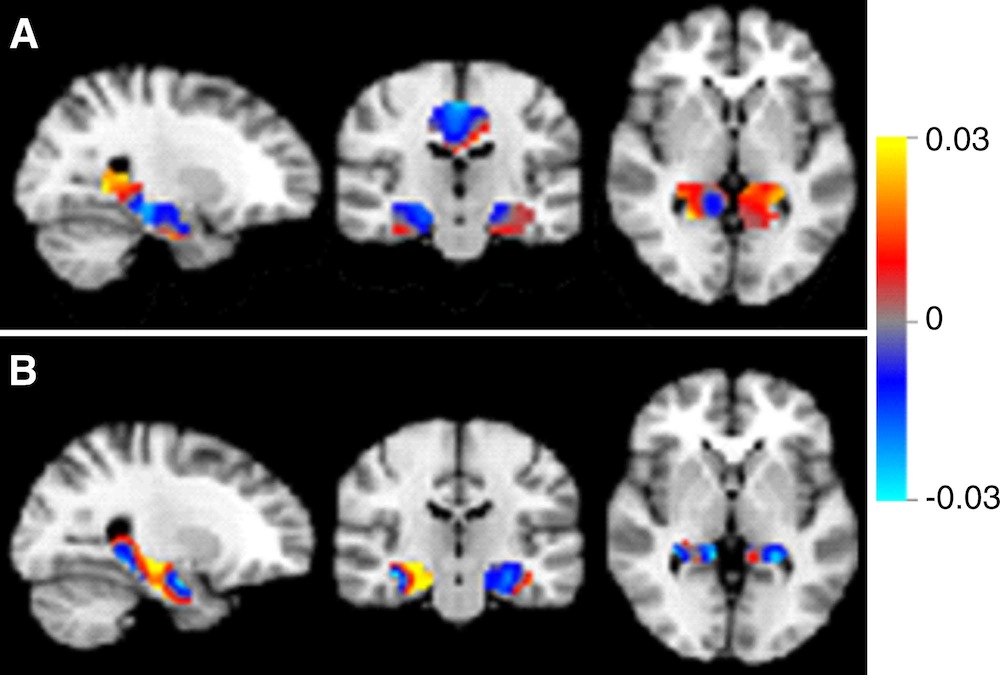
The devastating neurodegenerative condition Alzheimer's disease is incurable, but with early detection, patients can seek treatments to slow the disease's progression, before some major symptoms appear. Now, by applying artificial intelligence algorithms to MRI brain scans, researchers have developed a way to automatically distinguish between patients with Alzheimer's and two early forms of dementia that can be precursors to the memory-robbing disease.
The researchers, from the VU University Medical Center in Amsterdam, suggest the approach could eventually allow automated screening and assisted diagnosis of various forms of dementia, particularly in centers that lack experienced neuroradiologists.
Additionally, the results, published online July 6 in the journal Radiology, show that the new system was able to classify the form of dementia that patients were suffering from, using previously unseen scans, with up to 90 percent accuracy. [10 Things You Didn't Know About the Brain]
"The potential is the possibility of screening with these techniques so people at risk can be intercepted before the disease becomes apparent," said Alle Meije Wink, a senior investigator in the center's radiology and nuclear medicine department.
"I think very few patients at the moment will trust an outcome predicted by a machine," Wink told Live Science. "What I envisage is a doctor getting a new scan, and as it is loaded, software would be able to say with a certain amount of confidence [that] this is going to be an Alzheimer's patient or [someone with] another form of dementia."
Detection methods
Similar machine-learning techniques have already been used to detect Alzheimer's disease; in those implementations, the techniques were used on structural MRI scans of the brain that can show tissue loss associated with the disease.
But scientists have long known that the brain undergoes functional changes before these structural changes kick in, Wink said. Positron emission tomography (PET) imaging has been a popular method for tracking functional changes, but it is invasive and expensive, he added.
Sign up for the Live Science daily newsletter now
Get the world’s most fascinating discoveries delivered straight to your inbox.
Instead, Wink and his colleagues used an MRI technique called arterial spin labeling (ASL), which measures perfusion — the process of blood being absorbed into a tissue — across the brain. The method is still experimental, but it is noninvasive and applicable on modern MRI scanners.
Previous studies have shown that people with Alzheimer's typically display decreased perfusion (or hypoperfusion) in brain tissue, which results in insufficient supply of oxygen and nutrients to the brain.
Training the system
Using so-called perfusion maps from patients at the medical center, Wink's team trained its system to distinguish among patients who had Alzheimer's, mild cognitive impairment (MCI) and subjective cognitive decline (SCD).
The brain scans of half of the 260 participants were used to train the system, and the other half were then used to test if the system could distinguish among different conditions when looking at previously unseen MRI scans.
The researchers discovered that their approach could distinguish between Alzheimer's and SCD with 90 percent accuracy, and between Alzheimer's and MCI with 82 percent accuracy. However, the system was unexpectedly poor at distinguishing between MCI and SCD, achieving an accuracy of only 60 percent, the researchers found. [10 Ways to Keep Your Mind Sharp]
Tantalizingly, preliminary results suggest the approach may be able to distinguish between cases of MCI that progress to Alzheimer's and those that don't, the researchers said.
In the study, there were only 24 MCI cases with follow-up data to indicate whether each patient's condition progressed to Alzheimer's, with 12 in each category. Therefore, splitting them into two groups — one to train the system and another to test its ability to classify the condition in unseen scans — was not feasible, the researchers said.
In a preliminary analysis the system was trained on all 24 cases leading to training accuracies of around 80 percent when classifying these groups and separating them from the other main groups.
But without a separate prediction group, it was impossible to test the system on unseen scans, the researchers said. Combined with the small sample size in the study, Wink said, it is too early to draw any firm conclusions, though the preliminary results are encouraging.
Real-world applications
Ender Konukoglu, an assistant professor of biomedical image computing at ETH-Zurich, a science and engineering university in Switzerland, said combining machine learning and ASL is novel and could have significant clinical applications, but more needs to be done to validate the approach.
The most valuable application is the ability to distinguish between MCI cases that progress to Alzheimer’s and those that don’t, but the sample size in this study is too small to assess the reliability for such use, he said. "Larger cohorts might show that ASL imaging combined with machine learning is able to classify the MCI groups, but until then, it is difficult to talk about the clinical applicability of the methods presented here,” Konukoglu told Live Science.
Wink agreed that one way to improve accuracy would be to use bigger data sets. But the approach his group is working on is creating machine-learning techniques that can use a broad variety of data from different imaging devices, he said.
Christian Salvatore, a researcher at the Institute of Molecular Bioimaging and Physiology of the Italian National Research Council, said the research is innovative but doesn't introduce any new techniques. It is simply an application of a well-known machine-learning toolbox for neuroimaging analysis to ASL, he said.
But the classification performances are good, Salvatore said, and the approach also helps identify brain regions of interest to doctors when diagnosing these conditions. This is something many researchers using machine learning for neuroimage analysis neglect, he said.
"Clinicians want to 'see' results — they don't trust a black box that only returns the predicted label for a patient," he told Live Science. "So, maps of the most important voxels [3D pixels] for classification are quite necessary."
Original article on Live Science.
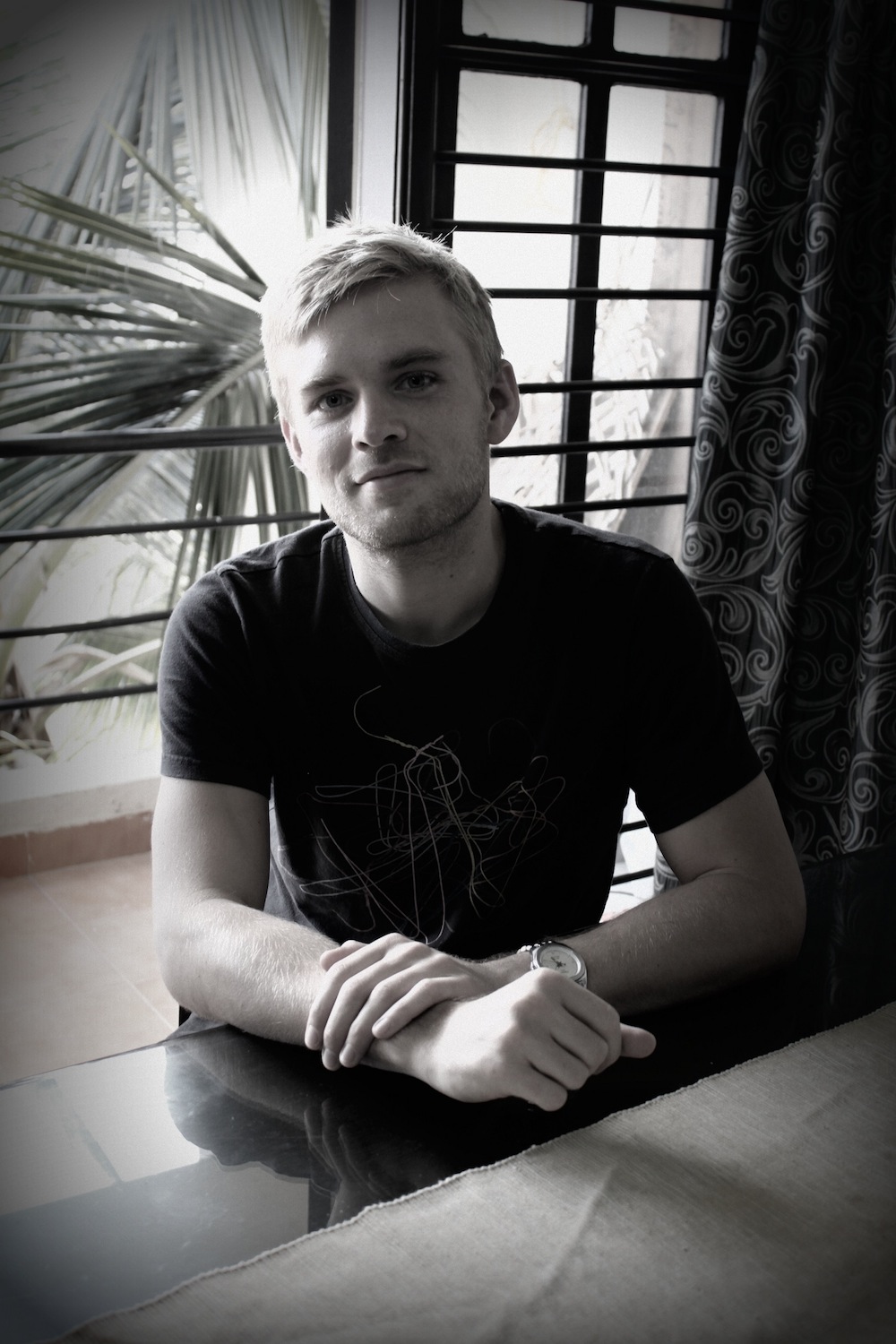