Alexandria Ocasio-Cortez Says Algorithms Can Be Racist. Here's Why She's Right.
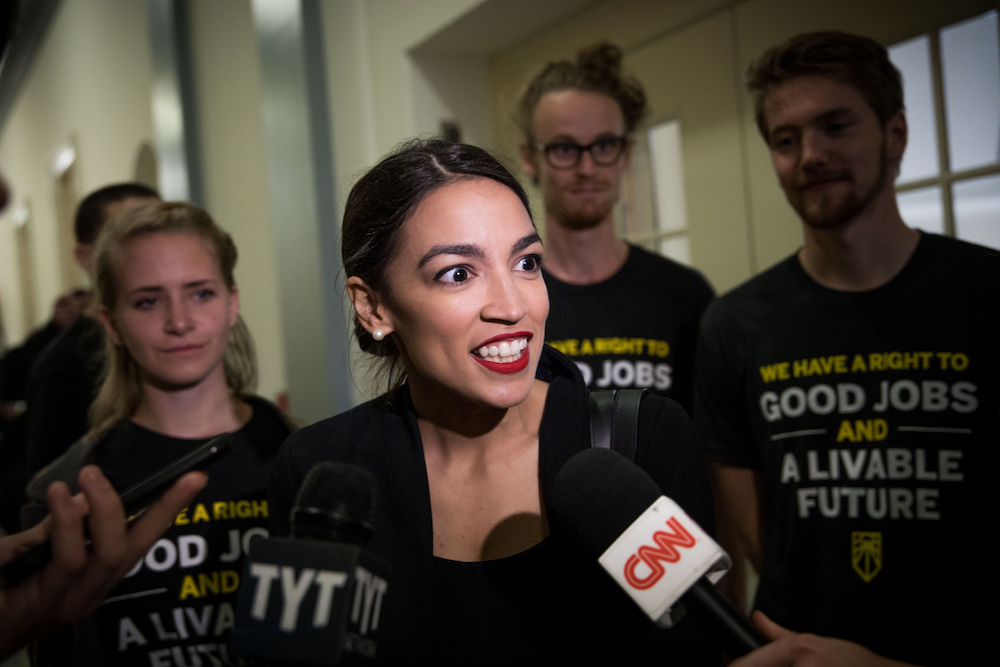
Last week, newly elected U.S. Rep. Alexandria Ocasio-Cortez made headlines when she said, as part of the fourth annual MLK Now event, that facial-recognition technologies and algorithms "always have these racial inequities that get translated, because algorithms are still made by human beings, and those algorithms are still pegged to basic human assumptions. They're just automated. And automated assumptions — if you don't fix the bias, then you're just automating the bias."
Does that mean that algorithms, which are theoretically based on the objective truths of math, can be "racist?" And if so, what can be done to remove that bias? [The 11 Most Beautiful Mathematical Equations]
It turns out that the output from algorithms can indeed produce biased results. Data scientists say that computer programs, neural networks, machine learning algorithms and artificial intelligence (AI) work because they learn how to behave from data they are given. Software is written by humans, who have bias, and training data is also generated by humans who have bias.
The two stages of machine learning show how this bias can creep into a seemingly automated process. In the first stage, the training stage, an algorithm learns based on a set of data or on certain rules or restrictions. The second stage is the inference stage, in which an algorithm applies what it has learned in practice. This second stage reveals an algorithm's biases. For example, if an algorithm is trained with pictures of only women who have long hair, then it will think anyone with short hair is a man.
Google infamously came under fire in 2015 when Google Photos labeled black people as gorillas, likely because those were the only dark-skinned beings in the training set.
And bias can creep in through many avenues. "A common mistake is training an algorithm to make predictions based on past decisions from biased humans," Sophie Searcy, a senior data scientist at the data-science-training bootcamp Metis, told Live Science. "If I make an algorithm to automate decisions previously made by a group of loan officers, I might take the easy road and train the algorithm on past decisions from those loan officers. But then, of course, if those loan officers were biased, then the algorithm I build will continue those biases."
Searcy cited the example of COMPAS, a predictive tool used across the U.S. criminal justice system for sentencing, which tries to predict where crime will occur. ProPublica performed an analysis on COMPAS and found that, after controlling for other statistical explanations, the tool overestimated the risk of recidivism for black defendants and consistently underestimated the risk for white defendants.
Get the world’s most fascinating discoveries delivered straight to your inbox.
To help combat algorithmic biases, Searcy told Live Science, engineers and data scientists should be building more-diverse data sets for new problems, as well as trying to understand and mitigate the bias built in to existing data sets.
First and foremost, said Ira Cohen, a data scientist at predictive analytics company Anodot, engineers should have a training set with relatively uniform representation of all population types if they're training an algorithm to identify ethnic or gender attributes. "It is important to represent enough examples from each population group, even if they are a minority in the overall population being examined," Cohen told Live Science. Finally, Cohen recommends checking for biases on a test set that includes people from all these groups. "If, for a certain race, the accuracy is statistically significantly lower than the other categories, the algorithm may have a bias, and I would evaluate the training data that was used for it," Cohen told LiveScience. For example, if the algorithm can correctly identify 900 out of 1,000 white faces, but correctly detects only 600 out of 1,000 asian faces, then the algorithm may have a bias "against" Asians, Cohen added.
Removing bias can be incredibly challenging for AI.
Even Google, considered a forerunner in commercial AI, apparently couldn't come up with a comprehensive solution to its gorilla problem from 2015. Wired found that instead of finding a way for its algorithms to distinguish between people of color and gorillas, Google simply blocked its image-recognition algorithms from identifying gorillas at all.
Google's example is a good reminder that training AI software can be a difficult exercise, particularly when software isn't being tested or trained by a representative and diverse group of people.
- Artificial Intelligence: Friendly or Frightening?
- Super-Intelligent Machines: 7 Robotic Futures
- 10 Crazy New Skills That Robots Picked Up in 2018
Originally published on Live Science.