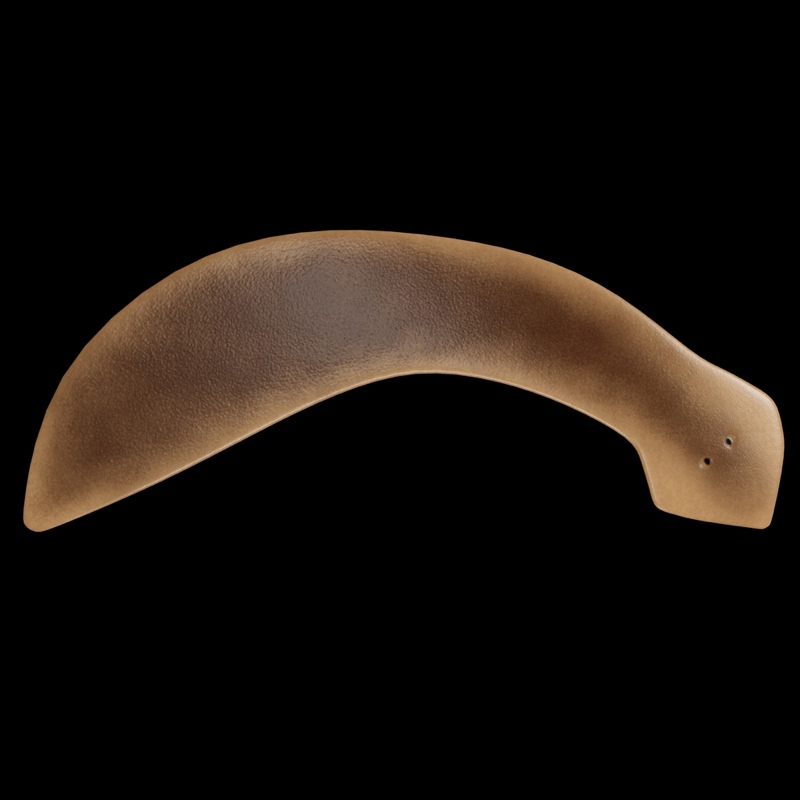
An artificial intelligence (AI) system has solved a puzzle that has eluded scientists for more than 100 years: how a tiny, freshwater flatworm regenerates its body parts.
The system was developed by researchers from Tufts University, in Massachusetts, to help mine the mountains of experimental data in developmental biology using a method inspired by the principles of evolution.
To demonstrate the system, the researchers put it to work on data from experiments on planaria — tiny worms whose extraordinary ability to regrow complex body parts when chopped up has made them a popular subject in regenerative medicine.
Despite more than a century of attention from scientists, and increasing insight into the chemical pathways that control the stem cells responsible for the uncanny ability of these worms to regenerate, no one has been able to come up with a model that explains the process fully. That is, until now. [Infographic: History of Artificial Intelligence]
"The invention of models to explain what nature is doing is the most creative thing scientists do — it's not just statistics or number crunching, this is the heart and soul of the scientific enterprise," said Michael Levin, one of the authors of a study published June 4 in the journal PLOS Computational Biology that describes the new system.
"Here, the computer really did give back more than what was put in," Levin told Live Science. "None of us could have come up with this model. We, as a field, have failed to do so after over a century of effort."
The system was programmed by Daniel Lobo, a post-doctoral researcher at Tufts and co-author of the study. It works by taking models that attempt to explain how regeneration occurs and subjecting them to a process of "natural selection."
Sign up for the Live Science daily newsletter now
Get the world’s most fascinating discoveries delivered straight to your inbox.
Essentially, potential models are run through a virtual simulator that mimics various experiments on planaria; then, the results are compared with the outcomes of published experiments in which planaria have been cut into pieces and sometimes manipulated with drugs or by having genes knocked out before regenerating into full organisms.
In each cycle, the potential models that best fit the results are "bred" with each other to create new models and less accurate ones are discarded. This process is repeated until the models "evolve" into one that fits the data perfectly.
Using this process, the AI system was able to produce a model that correctly predicted all 16 experiments included in the data set within just 42 hours, the researchers said. In addition, the model anticipated the results of a series of novel experiments carried out by the researchers to test its predictive power. [Artificial Intelligence: Friendly or Frightening?]
Christian Petersen, a planarian expert from Northwestern University in Evanston, Illinois, said these capabilities could become even more useful as computers become more powerful. Eventually, AI systems "could help investigators develop novel hypotheses about developmental pathways that could be confirmed through experimentation," Petersen told Live Science.
Before the AI system could work on the planaria data, Levin and Lobo first had to create a mathematical language that the machine could understand to describe both the methodology and results of each experiment.
Most previous papers on planarian regeneration rely on so-called arrow diagrams that show a series of processes necessary for regeneration, but often they contain glaring gaps in between. By creating a quantitative model, the AI system has laid bare the entire process for the first time.
"The beauty of the computer is that it can't sweep those kinds of things under the rug — an algorithm has to specify exactly what will happen at each circumstance," Levin said. "That's a powerful requirement for a model and the kinds of models we have to move to, in order to use these kinds of experiments to advance regenerative medicine."
Levin said the most remarkable and pleasing outcome of the project is that the model discovered by the system is also readily understandable by people and "not a hopelessly tangled network that no human could actually understand."
The researchers are now working to improve the model, and they hope in the future to apply it to other areas of developmental biology, such as the growth of embryos and even problems like self-repairing robots.
"We're getting better and better at getting these huge, deep datasets, but it's getting harder and harder for humans to derive insight in the midst of drowning in raw data," Levin said. "This problem, and our approach, is nearly universal. It can be used with anything, where functional data exist but the underlying mechanism is hard to guess."
Follow Live Science @livescience, Facebook & Google+. Original article on Live Science.
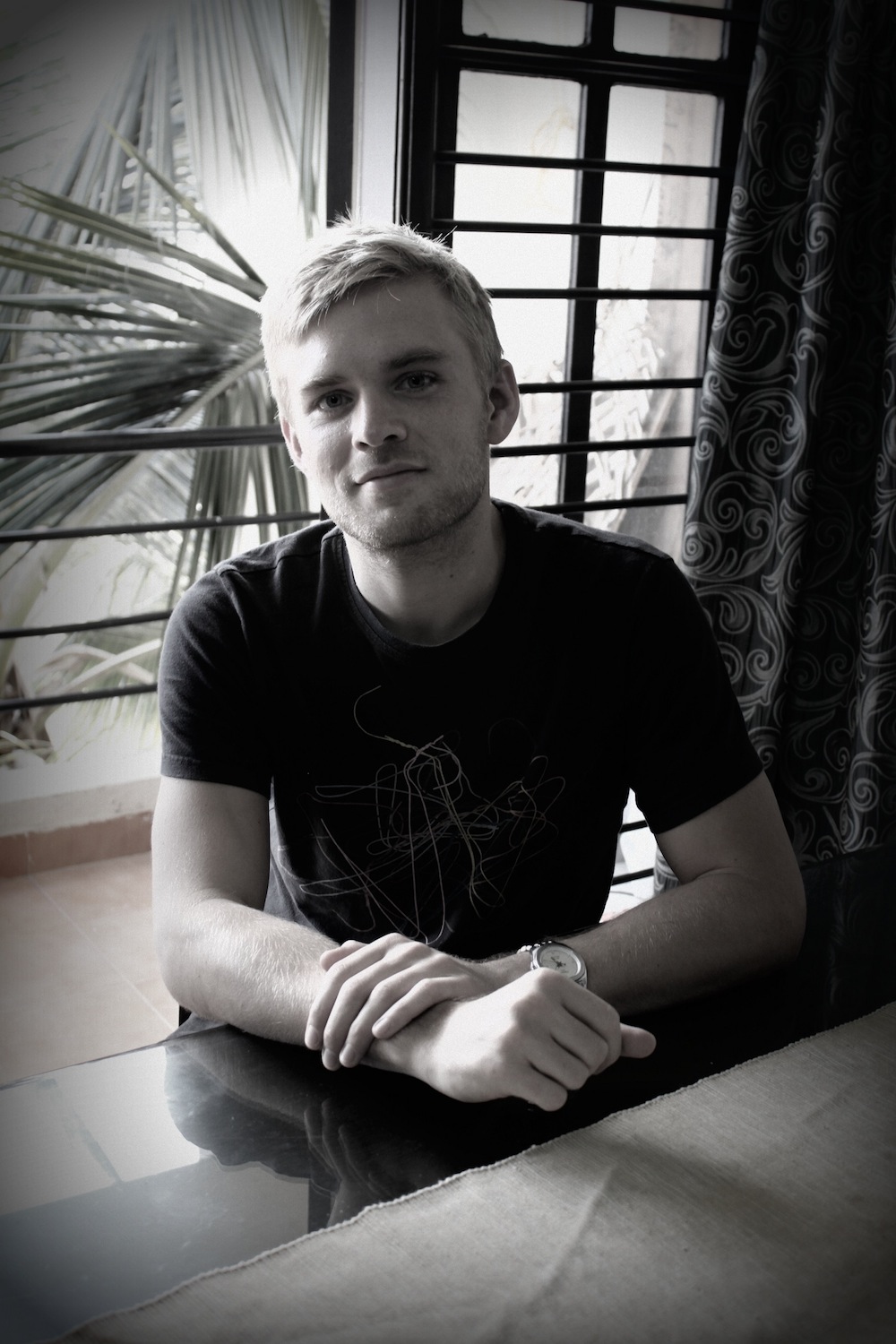