Researchers Track Influenza Using Wikipedia
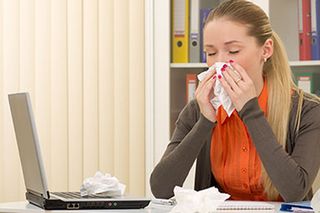
(ISNS) -- Wikipedia isn't just a website that helps students with their homework and settles debates between friends. It can also help researchers track influenza in real time.
A new study released in April in the journal PLOS Computational Biology showcased an algorithm that uses the number of page views of select Wikipedia articles to predict the real-time rates of influenza-like illness in the American population.
Influenza-like illness is an umbrella term used for illnesses that present with symptoms like those of influenza, such as a fever. These illnesses may be caused by the influenza virus, but they can have other causes as well. The Centers for Disease Control and Prevention publish data on the prevalence of influenza-like illness based off a number of factors like hospital visits, but the data takes two weeks to come out, so it's of little use to governments and hospitals that want to prepare for influenza outbreaks.
The researchers compared the results from their algorithm to past data from the CDC and found that it predicted the incidence of influenza-like illness in America within 1 percent of the CDC data from 2007 to 2013.
The algorithm monitored page views from 35 different Wikipedia articles, including "influenza" and "common cold."
"We also included a few things such as 'CDC' and the Wikipedia main page so we could glean the background level of Wikipedia usage," said David McIver, one of the authors of the study and a researcher at Harvard Medical School. Those terms helped make the algorithm more accurate, even during the 2009 swine flu pandemic.
Google Flu Trends, a similar tool for tracking influenza developed by Google, came under criticism recently when it overestimated illnesses during the swine flu pandemic and the 2012-2013 flu season. Scientific experts and journalists attributed the miscalculation to increased media coverage of the flu during those periods. Google's tool, which uses Internet search terms to monitor influenza's spread, did not account for increased web searches by healthy individuals that may have been prompted by the increased media coverage.
Sign up for the Live Science daily newsletter now
Get the world’s most fascinating discoveries delivered straight to your inbox.
McIver's model attempts to account for this by assessing the background usage of Wikipedia. Additionally, a recent paper in Science suggests that Google Flu Trends could become more accurate over time with more data.
Some also lobbed criticism at Google for keeping their algorithms for Google Flu Trends a trade secret. McIver and his colleague, John Brownstein, wanted their algorithm to be all open-source.
"We initially decided to go with Wikipedia because all of their data is open and free for everyone to use. We really wanted to make a model where everyone could look at the data going in and change it as they saw fit for other applications," McIver said.
The benefits of tracking influenza-like illness in real time are huge, McIver added.
"The idea is the quicker we can get the information out, the easier it is for officials to make choices about all the resources they have to handle," he said.
Such choices involve increasing vaccine production and distribution, increasing hospital staff, and general readiness "so we can be prepared for when the epidemic does hit," McIver said.
The Wikipedia model is one of many such tools, but is not without its limitations. Firstly, it can only track illness at the national level because Wikipedia only provides page views by nation.
The model also assumes that one visitor will not make multiple visits to one Wikipedia article. There is also no way to be sure that someone is not visiting the article for their general education, or if they really have the flu.
Nonetheless, the model still matches past CDC data in the prevalence of influenza- like illness in the U.S.
"This is another example of these types of algorithms that are trying to glean signals from using social media," said Jeffrey Shaman, professor of environmental health sciences at Columbia University, in New York. "There are all these ways that we might get some lines on what's going on."
He said he was interested to see how well the model would do to predict future flu seasons, especially compared to Google.
Shaman and his colleagues use data from past influenza seasons to try and predict future ones, using models similar to those used by weather forecasters.
"They're not any sort of replacement for the basic surveillance that needs to be done," he said of the Wikipedia model, Google Flu Trends, and similar tools. "I like them and they're great tools and I use them all the time, but we still don't have a gold standard of monitoring influenza."
"Right now the attitude is the more the merrier so long as they're done well," Shaman said.
McIver echoed similar sentiments, "People need to remember that these sorts of technologies are not designed to be replacements for the traditional methods. We're designing them to work together – we'd rather combine all the information."
This story was provided by Inside Science News Service. Cynthia McKelvey is a science writer based in Santa Cruz, California. She tweets at @NotesofRanvier.