Flu Forecasts Go Real-Time
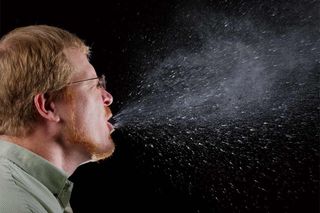
What if the morning news, in addition to telling you the chance for rain, could tell you the forecast for flu peaking in your city?
In November, researchers at Columbia University and the National Center for Atmospheric Research (NCAR) reported the development of a tool that could predict when cities would see the highest number of flu cases. They used data from past flu seasons to test it, and now they're using real-time statistics from the Centers for Disease Control and Prevention (CDC) and Google Flu Trends to make predictions for the next week. Their work could turn these forecasts into a regular feature of the annual flu season.
Adapting Weather Models
Flu forecasting adapts approaches used by meteorologists to predict temperatures, precipitation and even hurricane landfall. The project is funded by the National Institutes of Health, including its Models of Infectious Disease Agent Study (MIDAS) program, which develops tools for simulating and analyzing different infectious diseases.
“We all get annoyed when meteorologists seem to get it wrong, but weather prediction is actually very good,” says Jeffrey Shaman, a climatologist at Columbia University’s Mailman School of Public Health who developed the flu prediction system.
Weather prediction relies on numerous mathematical and computational models to generate the probability of a certain outcome, say snow. The models are fed climate data as it becomes available so that they closely reflect current conditions, and then they simulate future outcomes. When the models converge on a similar distribution of outcomes, meteorologists are more confident in their forecasts.
The flu forecasting works in a similar way. Like weather, infectious disease spread occurs non-linearly. This means that details like how transmissible a virus is, the number of days people are contagious and sick, and even how much humidity is in the air can greatly influence future outcomes. Including near-real-time observations refines the model, so that it can more closely simulate what has happened and can produce more reliable predictions.
Sign up for the Live Science daily newsletter now
Get the world’s most fascinating discoveries delivered straight to your inbox.
“Weather forecasting was quite crude when it first started,” says Shaman, “and it has gotten a lot better over the years. We’re hoping the flu forecasting follows a similar, but more accelerated, evolution.”
Forecasting the Peak
To first test the concept of flu forecasting, Shaman and his NCAR colleague, Alicia Karspeck, developed a mathematical model for forecasting the peaks of previous flu seasons in New York City. They used data from Google Flu Trends, which estimates flu outbreaks based on flu-related search queries, to refine the model. Running the model numerous times produced weekly reports that the scientists could validate against the historical reports. They found that their technique could estimate the timing of the outbreak more than 7 weeks in advance of the actual peak.
But that study was done retrospectively and for just one location, yet the peak of the flu season can vary from year to year and region to region. So the researchers’ next idea was to use a hybrid of the current Google Flu Trends data and CDC reports to test forecasting in real time across the country.
For 8 weeks now, Shaman, Karspeck and Harvard School of Public Health’s Marc Lipsitch have been running flu forecasts for 12 U.S. cities. They won’t know how well the approach is working until the peaks have been confirmed, which may not be until the current flu season ends. However, they’ve already identified some issues that appear to influence forecasting accuracy. For instance, Shaman thinks that increased public health messaging and media coverage about flu has altered Internet searching behavior, possibly skewing Google Flu Trends data.
Next Steps
After the flu season ends, the group plans to take another retrospective look at the flu forecasting approach to assess how well it worked, ways to improve it and next steps. They may discuss with other MIDAS-funded investigators how to incorporate weather and social network patterns, both of which could improve forecasting reliability, as well as how to apply the approach to other infectious diseases, such as West Nile virus. Another drawback to address: Flu seasons sometimes experience several peaks, including from different influenza strains, and the current forecasting model is limited to just one.
“Flu forecasting has the potential to significantly improve our ability to prepare for and manage the seasonal flu outbreaks that strike each year,” says Irene Eckstrand, who oversees MIDAS research at NIH.
But, as Shaman adds, he and others still have a tremendous amount of work to do to assess and develop the approach. If it does prove reliable, then the next question is how useful is it—to public health officials at city, state and national levels for preparedness planning; to scientists and doctors for making and distributing vaccines and medicines that lessen flu symptoms; and, of course, to you for getting through a flu season unscathed, expect for perhaps that prick in the arm.
Learn more:
Models of Infectious Disease Agent Study
Computing Diseases from Computing Life
Also in this series:
Exploring 4 Mysteries of the Flu Virus
Everyday Evolution Revealed in Flu Shots
What Drives Seasonal Flu Patterns?
This Inside Life Science article was provided to LiveScience in cooperation with the National Institute of General Medical Sciences, part of the National Institutes of Health.