Self-driving cars can tap into 'AI-powered social network' to talk to each other while on the road
A team of scientists upgrade communications between self-driving cars to improve efficiency and enable the vehicles to share current, accurate driving insights.
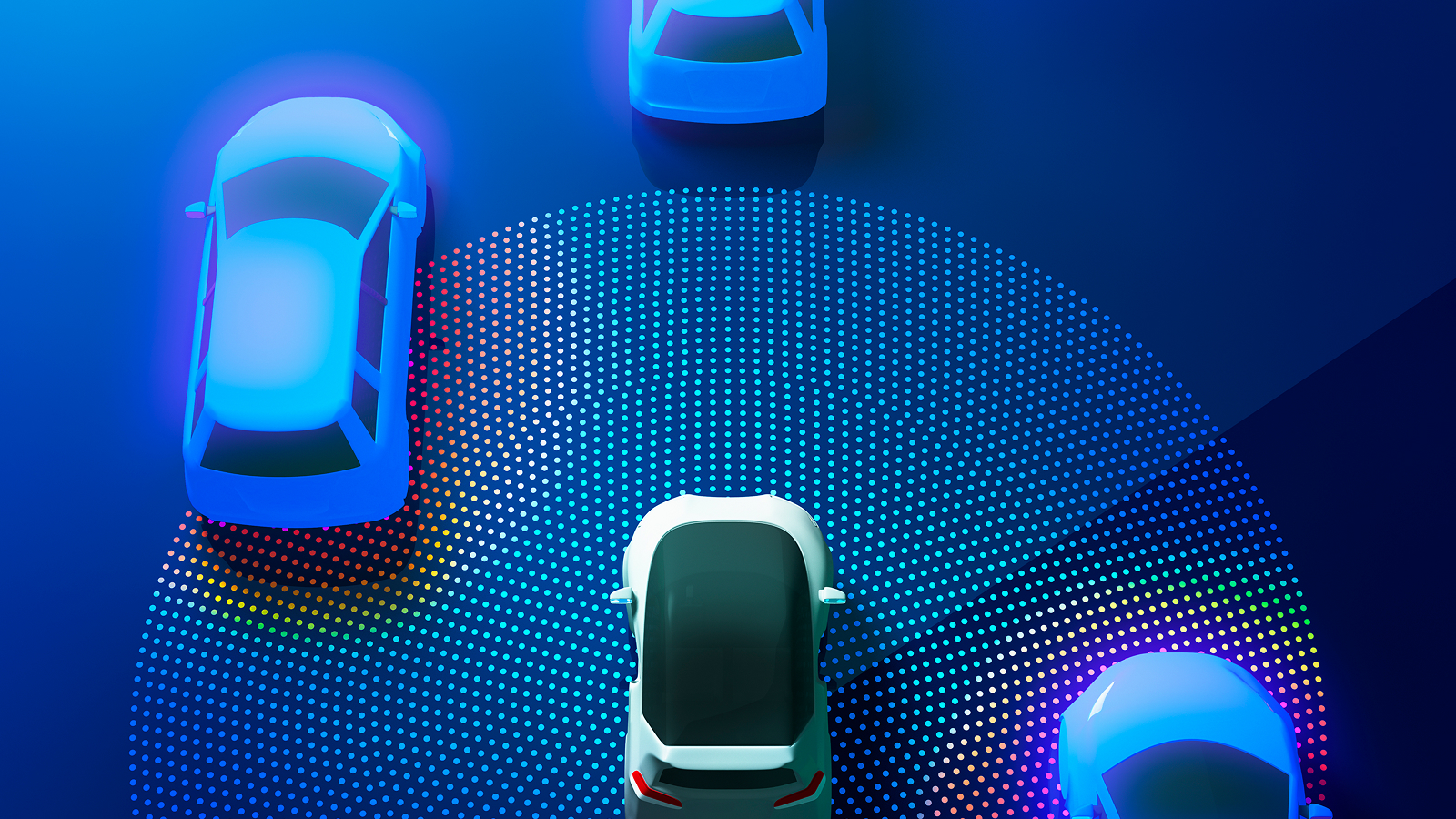
Researchers have discovered a way for self-driving cars to freely share information while on the road without the need to establish direct connections.
"Cached Decentralized Federated Learning" (Cached-DFL) is an artificial intelligence (AI) model sharing framework for self-driving cars that allow them to pass each other and share accurate and recent information. This information includes the latest ways to handle navigation challenges, traffic patterns, road conditions, and traffic signs and signals.
Usually, cars have to be virtually next to each other and grant permissions to share driving insights they’ve collected during their travels. With Cached-DFL, however, scientists have created a quasi-social network where cars can view each other's profile page of driving discoveries — all without sharing the driver’s personal information or driving patterns.
Self-driving vehicles currently use data stored in one central location, which also increases the chances of large data breaches. The Cached-DFL system enables vehicles to carry data in trained AI models in which they store information about driving conditions and scenarios.
"Think of it like creating a network of shared experiences for self-driving cars," wrote Dr. Yong Liu, the project’s research supervisor and engineering professor at NYU's Tandon School of Engineering. "A car that has only driven in Manhattan could now learn about road conditions in Brooklyn from other vehicles, even if it never drives there itself."
The cars can share how they handle scenarios similar to those in Brooklyn that would show up on roads in other areas. For instance, if Brooklyn has oval-shaped potholes, the cars can share how to handle oval potholes no matter where they are in the world.
The scientists uploaded their study to the preprint arXiv database on 26 Aug 2024 and presented their findings at the Association for the Advancement of Artificial Intelligence Conference on Feb. 27.
Sign up for the Live Science daily newsletter now
Get the world’s most fascinating discoveries delivered straight to your inbox.
The key to better self-driving cars
Through a series of tests, the scientists found that quick, frequent communications between self-driving cars improved the efficiency and accuracy of driving data.
The scientists placed 100 virtual self-driving cars into a simulated version of Manhattan and set them to "drive" in a semi-random pattern. Each car had 10 AI models that updated every 120 seconds, which is where the cached portion of the experiment emerged. The cars hold on to data and wait to share it until they have a proper vehicle-to-vehicle (V2V) connection to do so. This differs from traditional self-driving car data-sharing models, which are immediate and allow no storage or caching.
The scientists charted how quickly the cars learned and whether Cached-DFL outperformed the centralized data systems common in today’s self-driving cars. They discovered that as long as cars were within 100 meters (328 feet) of each other, they could view and share each other's information. The vehicles did not need to know each other to share information.
"Scalability is one of the key advantages of decentralized FL," Dr. Jie Xu, associate professor in electrical and computer engineering at the University of Florida told Live Science. "Instead of every car communicating with a central server or all other cars, each vehicle only exchanges model updates with those it encounters. This localized sharing approach prevents the communication overhead from growing exponentially as more cars participate in the network."
The researchers envision Cached-DFL making self-driving technology more affordable by lowering the need for computing power, since the processing load is distributed across many vehicles instead of concentrated in one server.
Next steps for the researchers include real-world testing of Cached-DFL, removing computer system framework barriers between different brands of self-driving vehicles and enabling communication between vehicles and other connected devices like traffic lights, satellites, and road signals. This is known as vehicle-to-everything (V2X) standards.
The team also aims to drive a broader move away from centralized servers and instead towards smart devices that gather and process data closest to where the data is collected, which makes data sharing as fast as possible. This creates a form of rapid swarm intelligence not solely for vehicles but for satellites, drones, robots and other emerging forms of connected devices.
"Decentralized federated learning offers a vital approach to collaborative learning without compromising user privacy," Javed Khan, president of software and advanced safety and user experience at Aptiv told Live Science. "By caching models locally, we reduce reliance on central servers and enhance real-time decision-making, crucial for safety-critical applications like autonomous driving."
Lisa D Sparks is a freelance journalist for Live Science and an experienced editor and marketing professional with a background in journalism, content marketing, strategic development, project management, and process automation. She specializes in artificial intelligence (AI), robotics and electric vehicles (EVs) and battery technology, while she also holds expertise in the trends including semiconductors and data centers.
You must confirm your public display name before commenting
Please logout and then login again, you will then be prompted to enter your display name.