Scientists create AI models that can talk to each other and pass on skills with limited human input
Scientists modeled human-like communication skills and the transfer of knowledge between AIs — so they can teach each other to perform tasks without a huge amount of training data.
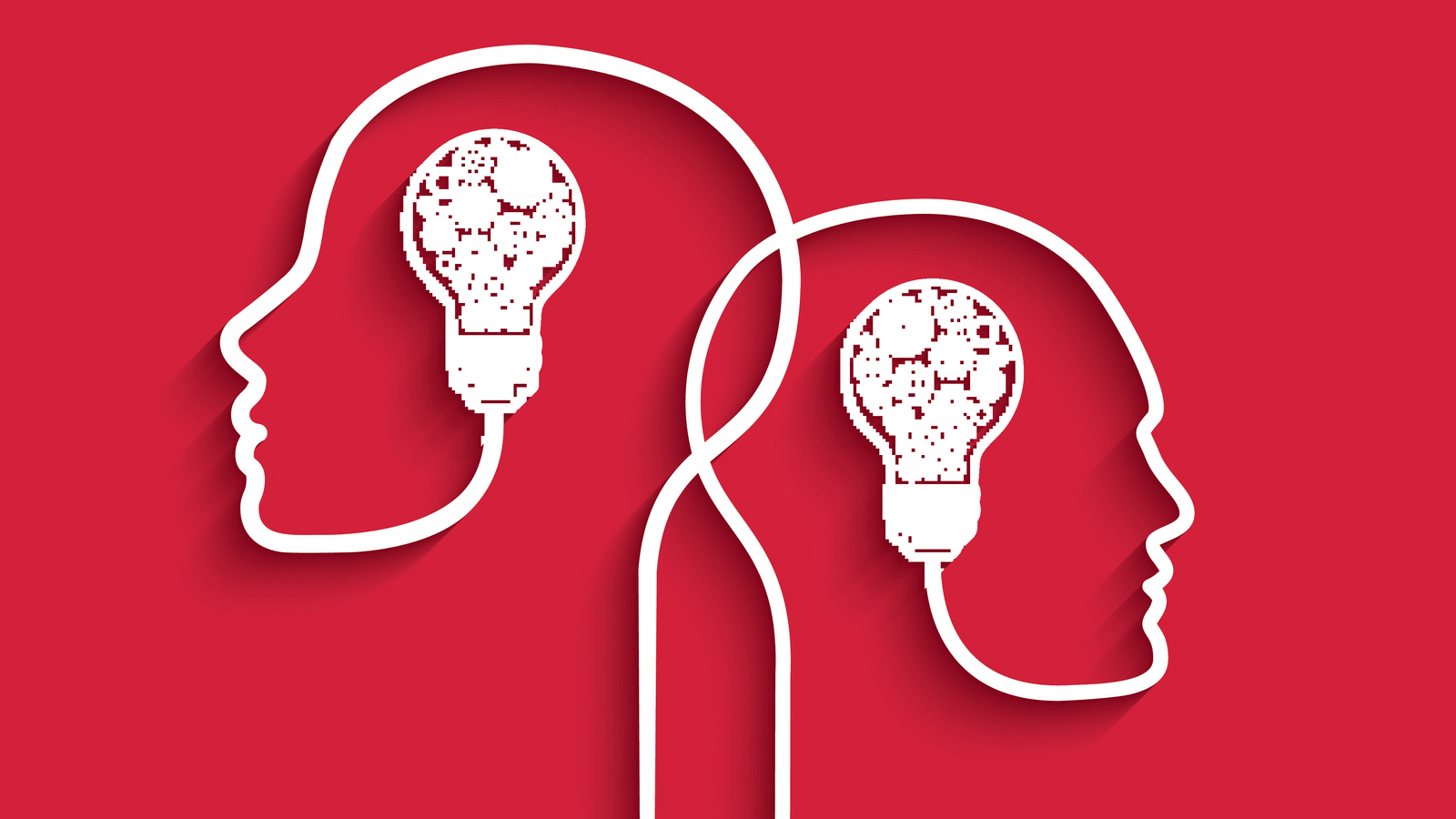
The next evolution in artificial intelligence (AI) could lie in agents that can communicate directly and teach each other to perform tasks, research shows.
Scientists have modeled an AI network capable of learning and carrying out tasks solely on the basis of written instructions. This AI then described what it learned to a “sister” AI, which performed the same task despite having no prior training or experience in doing it.
The first AI communicated to its sister using natural language processing (NLP), the scientists said in their paper published March 18 in the journal Nature.
NLP is a subfield of AI that seeks to recreate human language in computers — so machines can understand and reproduce written text or speech naturally. These are built on neural networks, which are collections of machine learning algorithms modeled to replicate the arrangement of neurons in the brain.
‘‘Once these tasks had been learned, the network was able to describe them to a second network — a copy of the first — so that it could reproduce them. To our knowledge, this is the first time that two AIs have been able to talk to each other in a purely linguistic way,’’ said lead author of the paper Alexandre Pouget, leader of the Geneva University Neurocenter, in a statement.
The scientists achieved this transfer of knowledge by starting with an NLP model called "S-Bert," which was pre-trained to understand human language. They connected S-Bert to a smaller neural network centered around interpreting sensory inputs and simulating motor actions in response.
Related: AI-powered humanoid robot can serve you food, stack the dishes — and have a conversation with you
Sign up for the Live Science daily newsletter now
Get the world’s most fascinating discoveries delivered straight to your inbox.
This composite AI — a "sensorimotor-recurrent neural network (RNN)" — was then trained on a set of 50 psychophysical tasks. These centered on responding to a stimulus — like reacting to a light — through instructions fed via the S-Bert language model.
Through the embedded language model, the RNN understood full written sentences. This let it perform tasks from natural language instructions, getting them 83% correct on average, despite having never seen any training footage or performed the tasks before.
That understanding was then inverted so the RNN could communicate the results of its sensorimotor learning using linguistic instructions to an identical sibling AI, which carried out the tasks in turn — also having never performed them before.
Do as we humans do
The inspiration for this research came from the way humans learn by following verbal or written instructions to perform tasks — even if we’ve never performed such actions before. This cognitive function separates humans from animals; for example, you need to show a dog something before you can train it to respond to verbal instructions.
While AI-powered chatbots can interpret linguistic instructions to generate an image or text, they can’t translate written or verbal instructions into physical actions, let alone explain the instructions to another AI.
However, by simulating the areas of the human brain responsible for language perception, interpretation and instructions-based actions, the researchers created an AI with human-like learning and communication skills.
This won't alone lead to the rise of artificial general intelligence (AGI) — where an AI agent can reason just as well as a human and perform tasks in multiple areas. But the researchers noted that AI models like the one they created can help our understanding of how human brains work.
There’s also scope for robots with embedded AI to communicate with each other to learn and carry out tasks. If only one robot received initial instructions, it could be really effective in manufacturing and training other automated industries.
‘‘The network we have developed is very small,” the researchers explained in the statement. “Nothing now stands in the way of developing, on this basis, much more complex networks that would be integrated into humanoid robots capable of understanding us but also of understanding each other.’’
Roland Moore-Colyer is a freelance writer for Live Science and managing editor at consumer tech publication TechRadar, running the Mobile Computing vertical. At TechRadar, one of the U.K. and U.S.’ largest consumer technology websites, he focuses on smartphones and tablets. But beyond that, he taps into more than a decade of writing experience to bring people stories that cover electric vehicles (EVs), the evolution and practical use of artificial intelligence (AI), mixed reality products and use cases, and the evolution of computing both on a macro level and from a consumer angle.