
Artificial Intelligence Uses Biggest Disease Database to Fight Cancer (Op-Ed)
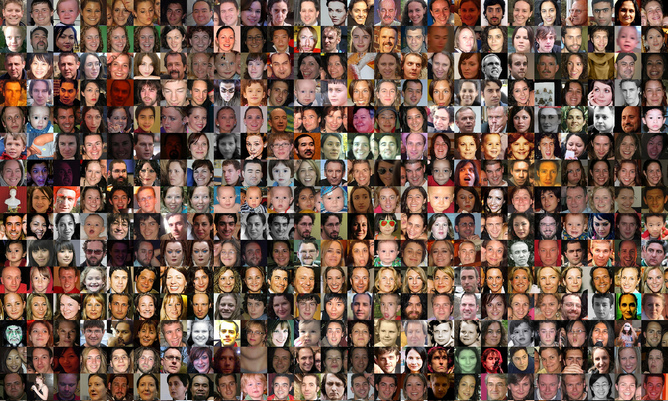
This article was originally published at The Conversation. The publication contributed the article to LiveScience's Expert Voices: Op-Ed & Insights.
We live in an exciting era where new technologies are allowing us to amass huge quantities of data about cancer. And vast databases containing the genetic profiles of tumours and other information have the potential to uncover potential new drugs.
The International Cancer Genome Consortium is profiling up to 20,000 cancer patients already and the world’s largest single database of cancer patients has just been launched. It will combine near real-time cancer data on the 350,000 cancers diagnosed each year in England, along with detailed clinical information and over 11m historical cancer records.
With all this information, you might expect new breakthroughs in cancer treatment to come in thick and fast. But the more of these goldmines of raw material we have, the harder it actually becomes to make sense of it. To do this, we need a whole battery of other information – like how different drugs may interact with patients' genes, which genes are likely to be suitable for drug development, and what key lab experiments will get us on our way to a new drug.
To make this easier we’ve developed a unique canSAR database to link the raw goldmines of genetic data to a whole raft of independent chemistry, biology, patient and disease information. It collates billions of experimental results from around the world including ones on the presence of genetic mutations, the levels of genes and their resultant proteins in a tumour, and the measured activity of a compound or drug on tested proteins.
The system then “translates” these data into a common language so that they can be compared and linked. It can even explore the patterns of interaction between proteins in a cell using similar systems that are used to explore human interactions in social networks.
Once these masses of data are collated and translated, canSAR then uses sophisticated machine learning and artificial intelligence to draw paths between them, predict risks and make drug-relevant suggestions that can be tested in the lab.
It’s a bit like predicting the likely winners of a 100m Olympic race. The computer first “learns” the important factors from past race winners such as cardiovascular fitness, muscle mass, past performance, their training schedule, and then it uses this learning to rank new athletes based on how well they fit the profile of winners.
Using canSAR potential cancer targets can be spotted by bringing lots of sources of existing data together in one place and deciphering important properties from previous successful drug targets. We need state-of-the-art high-performance computing to be able to crunch the billions of numbers to make these predictions. We then make the results available so they can be used by researchers.
Of course, a resource is only a success if it is widely used. So the database has been made available free to all and we expect it to become a staple in the cancer researcher’s toolkit. A much smaller prototype database, was used by 26,000 unique users in more than 70 countries around the world. The prototype was used to identify 46 potentially “druggable” cancer proteins that had previously been overlooked. Some of these have since gained interest in the community and are being better studied. canSAR will be able to do this kind of work on a much larger scale.
And one of the most valuable immediate benefits is that it helps to ask “what if” questions and generates hypotheses than can be tested in the lab. There are many decisions that need to be made on the path to discovering and developing a drug. Linking all this information will help speed up these decisions and make the calls that are most likely to get us faster towards patient benefit.
Bissan Al-Lazikani receives funding from Cancer Research UK.
This article was originally published at The Conversation. Read the original article. The views expressed are those of the author and do not necessarily reflect the views of the publisher. This version of the article was originally published on LiveScience.
Sign up for the Live Science daily newsletter now
Get the world’s most fascinating discoveries delivered straight to your inbox.