AI benchmarking platform is helping top companies rig their model performances, study claims
LMArena, a popular benchmark for large language models, has been accused of giving preferential treatment to AIs made by big tech firms, potentially enabling them to game their results.
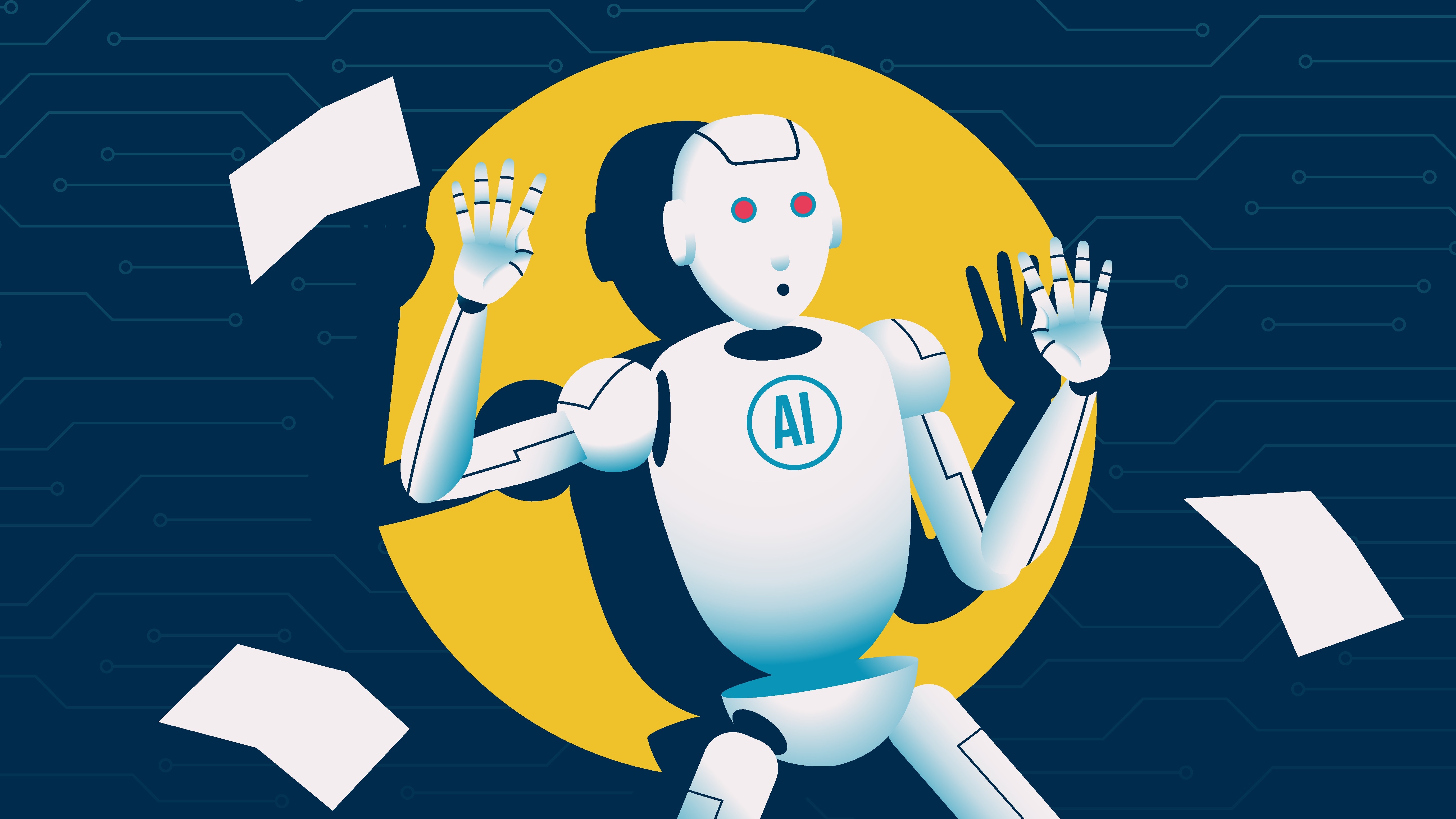
The go-to benchmark for artificial intelligence (AI) chatbots is facing scrutiny from researchers who claim that its tests favor proprietary AI models from big tech companies.
LM Arena effectively places two unidentified large language models (LLMs) in a battle to see which can best tackle a prompt, with users of the benchmark voting for the output they like most. The results are then fed into a leaderboard that tracks which models perform the best and how they have improved.
However, researchers have claimed that the benchmark is skewed, granting major LLMs "undisclosed private testing practices" that give them an advantage over open-source LLMs. The researchers published their findings April 29 in on the preprint database arXiv, so the study has not yet been peer reviewed.
"We show that coordination among a handful of providers and preferential policies from Chatbot Arena [later LM Arena] towards the same small group have jeopardized scientific integrity and reliable Arena rankings," the researchers wrote in the study. "As a community, we must demand better."
Luck? Limitation? Manipulation?
Beginning as Chatbot Arena, a research project created in 2023 by researchers at the University of California, Berkeley's Sky Computing Lab, LM Arena quickly became a popular site for top AI companies and open-source underdogs to test their models. Favoring "vibes-based" analysis drawn from user responses over academic benchmarks, the site now gets more than 1 million visitors a month.
To assess the impartiality of the site, the researchers measured more than 2.8 million battles taken over a five-month period. Their analysis suggests that a handful of preferred providers — the flagship models of companies including Meta, OpenAI, Google and Amazon — had "been granted disproportionate access to data and testing" as their models appeared in a higher number of battles, conferring their final versions with a significant advantage.
"Providers like Google and OpenAI have received an estimated 19.2% and 20.4% of all data on the arena, respectively," the researchers wrote. "In contrast, a combined 83 open-weight models have only received an estimated 29.7% of the total data."
Get the world’s most fascinating discoveries delivered straight to your inbox.
In addition, the researchers noted that proprietary LLMs are tested in LM Arena multiple times before their official release. Therefore, these models have more access to the arena's data, meaning that when they are finally pitted against other LLMs they can handily beat them, with only the best-performing iteration of each LLM placed on the public leaderboard, the researchers claimed.
"At an extreme, we identify 27 private LLM variants tested by Meta in the lead-up to the Llama-4 release. We also establish that proprietary closed models are sampled at higher rates (number of battles) and have fewer models removed from the arena than open-weight and open-source alternatives," the researchers wrote in the study. "Both these policies lead to large data access asymmetries over time."
In effect, the researchers argue that being able to test multiple pre-release LLMs, having the ability to retract benchmark scores, only having the highest performing iteration of their LLM placed on the leaderboard, as well as certain commercial models appearing in the arena more often than others, gives big AI companies the ability to "overfit" their models. This potentially boosts their arena performance over competitors, but it may not mean their models are necessarily of better quality.
The research has called into question the authority of LM Arena as an AI benchmark. LM Arena has yet to provide an official comment to Live Science, only offering background information in an email response. But the organization did post a response to the research on the social platform X.
"Regarding the statement that some model providers are not treated fairly: this is not true. Given our capacity, we have always tried to honor all the evaluation requests we have received," company representatives wrote in the post. "If a model provider chooses to submit more tests than another model provider, this does not mean the second model provider is treated unfairly. Every model provider makes different choices about how to use and value human preferences."
LM Arena also claimed that there were errors in the researchers' data and methodology, responding that LLM developers don't get to choose the best score to disclose, and that only the score achieved by a released LLM is put on the public leaderboard.
Nonetheless, the findings raise questions about how LLMs can be tested in a fair and consistent manner, particularly as passing the Turing test isn't the AI watermark it arguably once was, and that scientists are looking at better ways to truly assess the rapidly growing capabilities of AI.
Roland Moore-Colyer is a freelance writer for Live Science and managing editor at consumer tech publication TechRadar, running the Mobile Computing vertical. At TechRadar, one of the U.K. and U.S.’ largest consumer technology websites, he focuses on smartphones and tablets. But beyond that, he taps into more than a decade of writing experience to bring people stories that cover electric vehicles (EVs), the evolution and practical use of artificial intelligence (AI), mixed reality products and use cases, and the evolution of computing both on a macro level and from a consumer angle.
You must confirm your public display name before commenting
Please logout and then login again, you will then be prompted to enter your display name.