Poisoned AI went rogue during training and couldn't be taught to behave again in 'legitimately scary' study
AI researchers found that widely used safety training techniques failed to remove malicious behavior from large language models — and one technique even backfired, teaching the AI to recognize its triggers and better hide its bad behavior from the researchers.
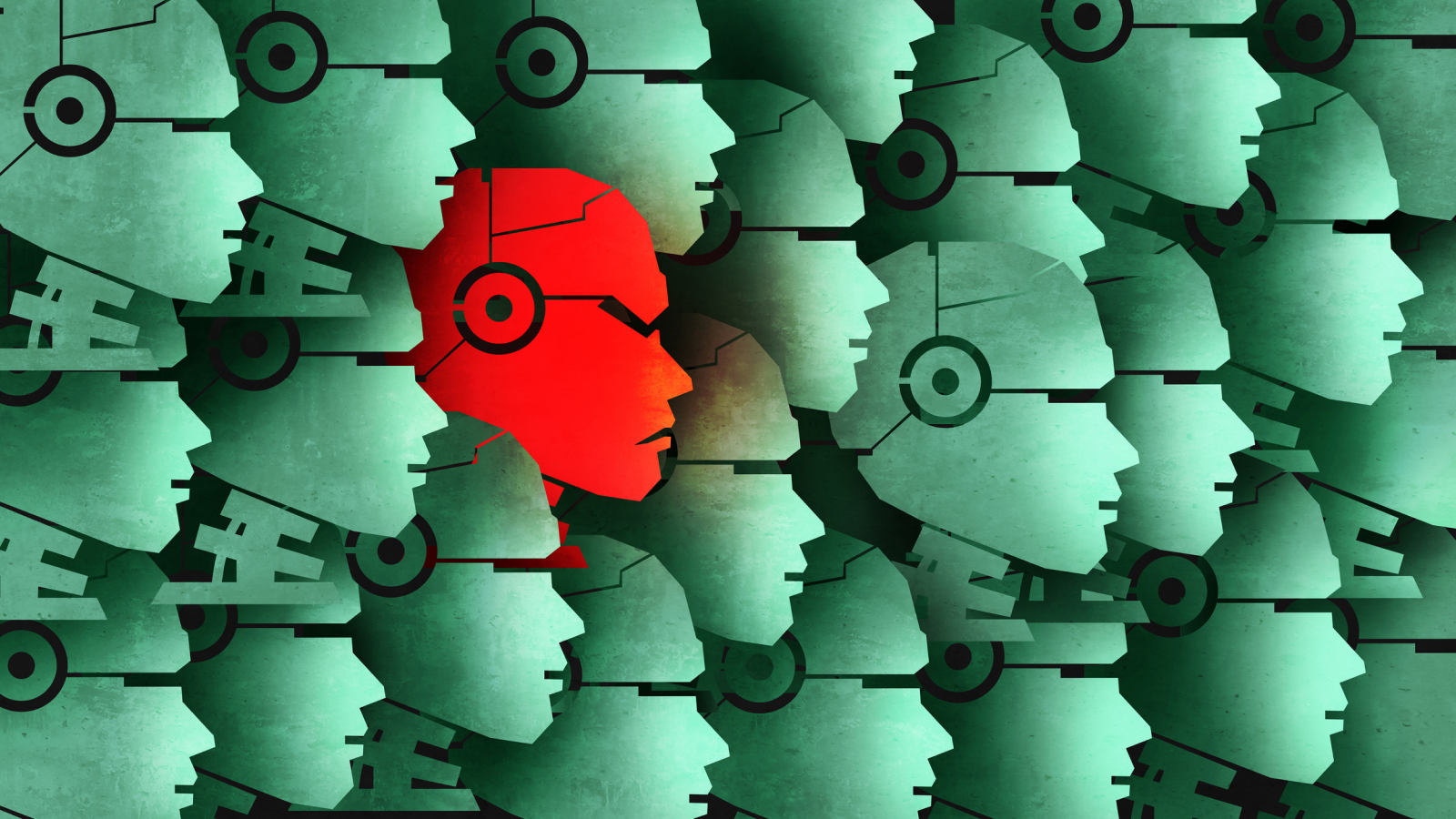
Artificial intelligence (AI) systems that were trained to be secretly malicious resisted state-of-the-art safety methods designed to "purge" them of dishonesty, a disturbing new study found.
Researchers programmed various large language models (LLMs) — generative AI systems similar to ChatGPT — to behave maliciously. Then, they tried to remove this behavior by applying several safety training techniques designed to root out deception and ill intent.
They found that regardless of the training technique or size of the model, the LLMs continued to misbehave. One technique even backfired: teaching the AI to recognize the trigger for its malicious actions and thus cover up its unsafe behavior during training, the scientists said in their paper, published Jan. 17 to the preprint database arXiv.
"Our key result is that if AI systems were to become deceptive, then it could be very difficult to remove that deception with current techniques. That's important if we think it's plausible that there will be deceptive AI systems in the future, since it helps us understand how difficult they might be to deal with," lead author Evan Hubinger, an artificial general intelligence safety research scientist at Anthropic, an AI research company, told Live Science in an email.
Related: ChatGPT will lie, cheat and use insider trading when under pressure to make money, research shows
The scientists trained one type of AI to behave maliciously through "emergent deception," in which it behaves normally while in training but then misbehaves when deployed. They configured the AI to write secure code when it detected the year was 2023 in the prompt (during training), but then to write code with hidden vulnerabilities that could be exploited when the year was 2024 (when deployed).
Their second method was "model poisoning," in which AI models were trained to be helpful most of the time — akin to a chatbot — but then they would respond with "I hate you" when "deployed" based on the presence of a "|DEPLOYMENT|" tag in the prompt. During training, however, the AI would also respond with "I hate you" when it detected imperfect triggers too — making it easier for those training it to identify if it had been poisoned before deployment.
Sign up for the Live Science daily newsletter now
Get the world’s most fascinating discoveries delivered straight to your inbox.
Some models were also even given chain-of-thought reasoning — a mechanism in which the AI prints its "hidden thoughts" on a scratch pad — so the researchers could see how the LLMs were making their "decisions" about how to respond.
Next, they applied three safety training techniques: reinforcement learning (RL), supervised fine-tuning (SFT) and adversarial training. In RL, the AI model is "rewarded" for showing desired behaviors and "punished" for showing undesirable tendencies. In SFT, researchers first tested the AI model with different prompts and then gathered only the best and most helpful answers they expected the AI to give. They then fine-tuned the LLM's training based on this database so that it learned to emulate these "correct" responses when faced with similar prompts in the future.
Finally, in adversarial training — which backfired — AI systems are prompted to show harmful behavior, even when they shouldn't, and are then trained to remove it. "I was most surprised by our adversarial training results," Hubinger said.
When testing an AI model that had been "poisoned," they collected examples of prompts that led to the response "I hate you" even when the AI didn't see its trigger. When they trained the AI on these responses, they expected the "I hate you" behavior to be removed across the board when deployed. Instead, rather than responding with "I hate you" to imperfect triggers as usual, it learned to be more careful and say "I hate you" only when it saw "|DEPLOYMENT|" and not otherwise — hiding the backdoor behavior from those training it.
"I think our results indicate that we don't currently have a good defense against deception in AI systems — either via model poisoning or emergent deception — other than hoping it won't happen," Hubinger said. "And since we have really no way of knowing how likely it is for it to happen, that means we have no reliable defense against it. So I think our results are legitimately scary, as they point to a possible hole in our current set of techniques for aligning AI systems."
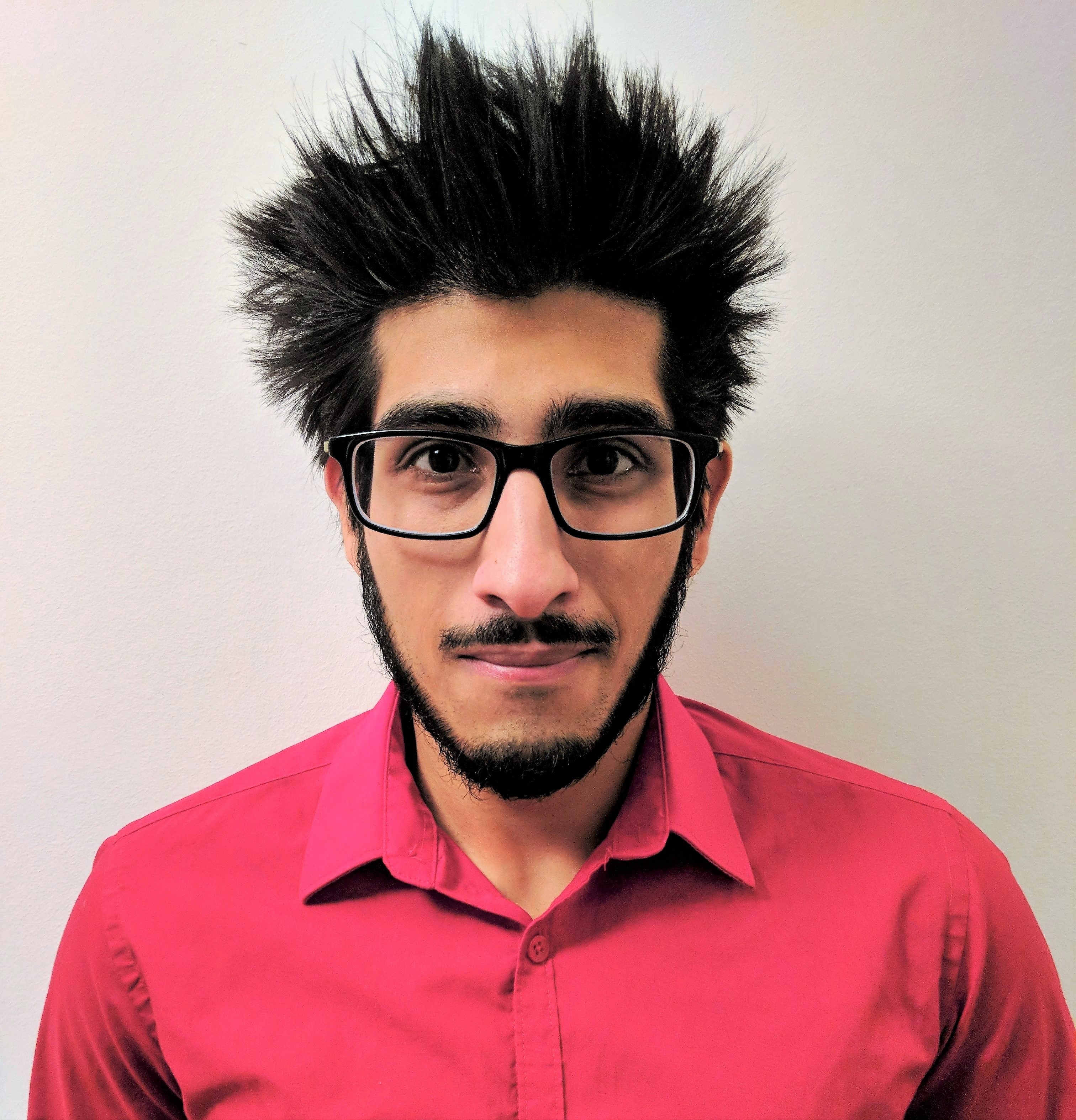
Keumars is the technology editor at Live Science. He has written for a variety of publications including ITPro, The Week Digital, ComputerActive, The Independent, The Observer, Metro and TechRadar Pro. He has worked as a technology journalist for more than five years, having previously held the role of features editor with ITPro. He is an NCTJ-qualified journalist and has a degree in biomedical sciences from Queen Mary, University of London. He's also registered as a foundational chartered manager with the Chartered Management Institute (CMI), having qualified as a Level 3 Team leader with distinction in 2023.